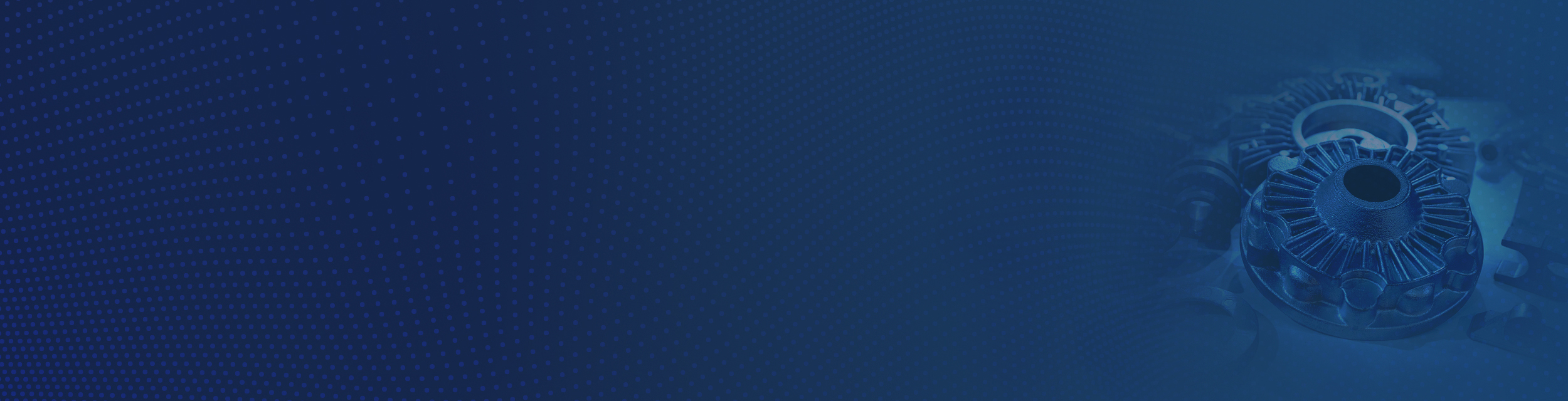
Up to 10 % energy cost savings
Data Driven Engineering
Using AI in the manufacturing process
Machine learning in mechanical engineering: Thanks to the use of machine learning methods for the early detection of casting defects, impressive results have already been achieved during the introduction into practical application.
Problem definition
In the world of casting production, the final quality of the products depends on a variety of process and machine parameters. Interestingly, solid components in particular are extremely sensitive to the various influencing factors during production. The problem lies not only in identifying the decisive process variables to ensure first-class end products, but also in the often lacking possibility of a final 100% quality inspection.
In the production processes, not only must measurable variables such as temperatures, cooling times, vibrations, environmental conditions and machine parameters be taken into account, but also the experience of the machine operators, who react to deviating conditions or visible deviations in the manufacturing process.
The digitalization of these practices offers promising prospects for the future. It should be noted that the two main areas, namely the dynamic measured variables and the subjective judgment of the employees, should not be viewed strictly separately. Rather, they should be considered as interlinked elements in order to increase the quality and efficiency of casting production.
Objective
In the production of castings, the challenge is that it is difficult to put into words the years of experience accumulated by machine operators and even more difficult to translate into clear programmable rules. This represents a barrier to the goal of digitalization and automated quality improvement and inspection.
The application of machine learning can help to utilize these existing data sources and transfer the team's valuable empirical knowledge into practicable structures. With these methods, an immense amount of process parameters can be evaluated simultaneously and predictions can be made about possible sources of error. This means that experienced experts are no longer forced to keep an eye on hundreds of parameters. Instead, they can concentrate on the key factors and intervene in the manufacturing process in a targeted manner.
The aim of this measure is to reduce production errors, be it due to inadequate cooling processes, belt speeds or fluctuations in the chemical composition of the base material. The aim is to detect these influences as early as possible during production in order to minimize follow-up costs due to faulty components. The aim is to use machine learning to optimize the future of casting production, drawing on the invaluable knowledge of employees.
Result
The implementation and integration of supporting machine learning algorithms took place immediately after completion of the technical foundations and initial training. The dynamics of these algorithms, which develop through continuous learning in the ongoing production process, provide future-oriented security and improved prediction capacities for future product lines of cast parts.
Even at the time of launch, even with limited training data, precise forecasts can be made regarding the expected quality of the parts to be produced. These analyses can be used to specifically identify individual parts in series production that are most likely to be defective, and they can either be examined more closely or excluded from the production chain. This enables short-term savings in the downstream production steps and covers the costs of the machine learning project.
The current level of knowledge and developments makes it much easier to monitor and control the process. However, as production series progress and the volume of training data increases, it will be possible to go even further. The focus here is on techniques that are suitable for series production, such as sonic investigations or image recordings, which, in conjunction with the machine learning algorithms, enable 100% non-destructive testing of the end products.
One key to success is the use of the experts' specialist knowledge to assess the end products. They are now able to intensively examine selected components instead of having to rely on random sample analyses to make statements about series production.
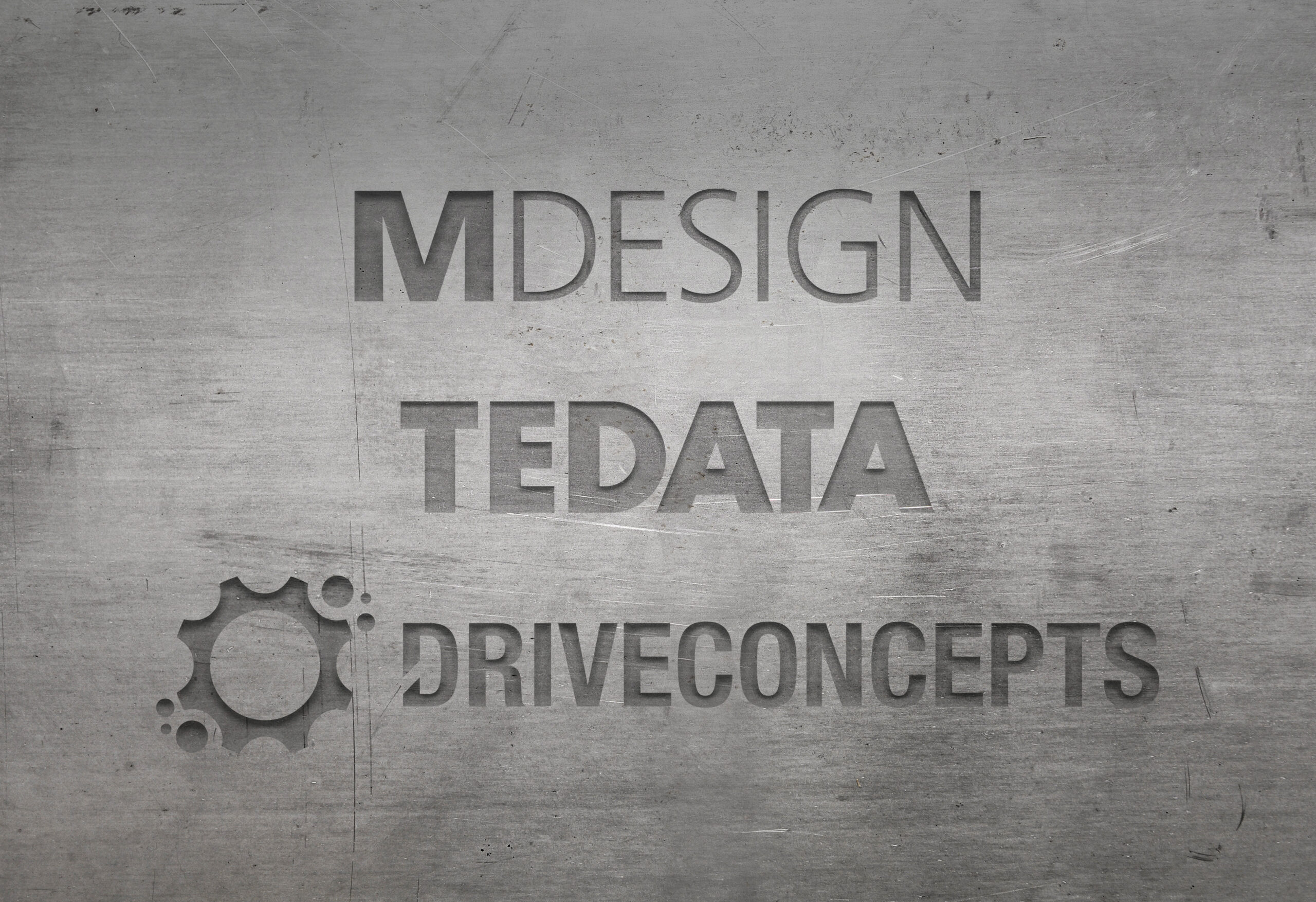
Knowledge for technology
Your strong partners in product development
We support industrial companies on their path to digital transformation. With customized services in simulation, calculation and software development, we promote efficient product development. Put your trust in over 40 years of experience and shape your industrial future.